Supply Chain Optimization
AI-led approach revamped route planning for a logistics provider, leading to better punctuality and lower fuel usage. They quickly outpaced competitors through improved efficiency and customer satisfaction.
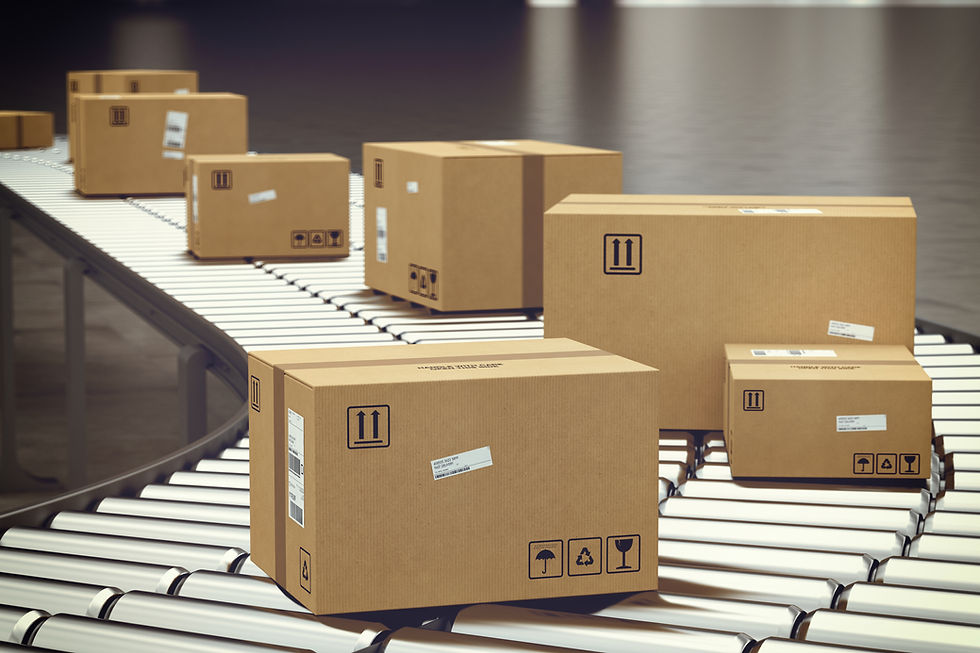
Traditional Challenges
Rigid Reorder Points: Static thresholds often result in overstock when demand dips—or empty shelves when demand spikes.
Manual Routing: Dispatchers map delivery runs by hand, missing opportunities to shave miles and time off each trip.
Siloed Visibility: Inventory, order, and supplier data live in separate systems, making it hard to spot bottlenecks before they bite.
Reactive Problem-Solving: Teams scramble to address delays or supplier issues after they occur, rather than preventing them.
AI-Driven Approach
Predictive Inventory Balancing
Process: Machine-learning models ingest sales history, seasonality, and promotional calendars to forecast demand at each location.
Outcome: Stock is automatically rebalanced across warehouses—cutting holding costs and reducing both overstock and stockouts.
Dynamic Route Planning
Process: AI engines analyze live traffic feeds, driver availability, and delivery windows.
Outcome: Daily delivery routes adjust in real time, minimizing fuel use and transit times while meeting customer promises.
Supplier Risk Monitoring
Process: Natural-language tools scan news, weather alerts, and trade-data feeds to assess supplier reliability.
Outcome: Procurement teams receive early warnings of potential disruptions—such as natural disasters or port strikes—so they can qualify alternates before orders stall.
Demand-Sensing Forecasts
Process: Advanced analytics blend point-of-sale data, social-media trends, and macroeconomic indicators to detect emerging demand shifts.
Outcome: Planning teams react proactively to surges or dips—avoiding panic orders or wasted production runs.
Key Benefits
Lower Holding Costs: Predictive balancing can cut warehouse inventory by 10–15% without raising stockout risk.
Faster Deliveries: Dynamic routing shrinks last-mile times by 20–25%, boosting customer satisfaction.
Improved Resilience: Supplier-risk alerts extend lead-time visibility by several weeks, preventing emergency sourcing.
Data-Driven Agility: End-to-end visibility turns reactive firefighting into proactive planning.
Real-World Example
A major e-commerce retailer struggled with seasonal spikes that left some regional warehouses overfilled and others empty. By deploying an AI inventory-balancing system, they automatically shifted stock between three sites based on forecasted sales patterns—reducing overall holding costs by 12% and slashing stockouts during peak season by 30%. Simultaneously, an AI routing platform reconfigured daily delivery runs, cutting average transit times by 22% and lowering fuel expenses. These combined gains translated into happier customers, leaner operations, and a more resilient supply chain.